|
Post by dividavi on Feb 15, 2020 1:19:36 GMT
For whatever reason there is great concern about wet sidewalks and how that may or may not address the stupidity of the adage, "Absence of evidence is not evidence of absence." I'll submit some photographs to demonstrate the truth of my statement that it's a silly saying. Instead of sidewalks, an urban phenomenon, I'll use the results of a google image search using rural road wet as key words.  This is from France and the road does appear to be shining wet. Did it rain at this location less than six hours before the photo was taken? No, because that's not how rain works. In this case the wet road is caused by snow, something different from rain.  Did it rain less than six hours before the above photo was taken? It's certain that it did although a dunderhead could say that rainfall has not been established. 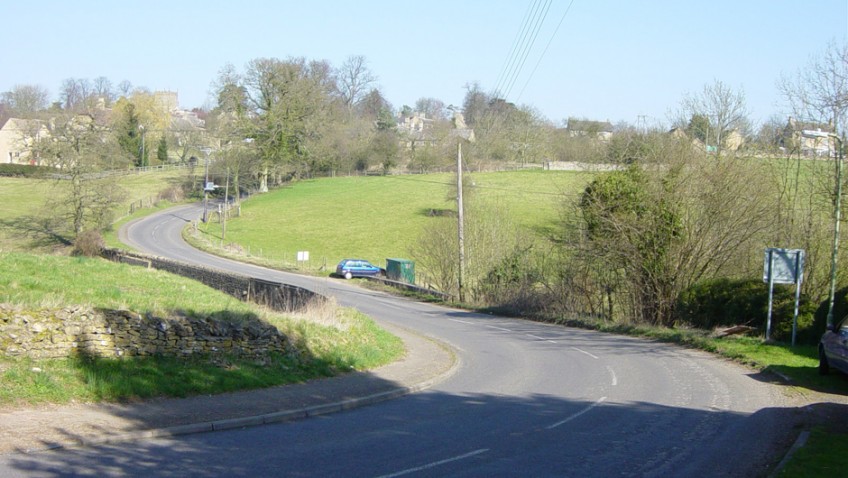 There's no evidence that it rained in the last six hours. Is that evidence that it did not rain in that period? Sure it is. If rain has fallen at any location there should be residual evidence of that occurrence for a few hours. The exact period varies with temperature and other factors. Similarly with a murder or some major crime one would expect that the perpetrator has left some traces that indicate he was involved in the crime. If no such traces are found to connect an individual to a particular crime it is usually the case that the person is not involved; somebody else did it. Absence of evidence is evidence (not proof, evidence) of absence.
|
|
|
Post by Arlon10 on Feb 15, 2020 1:31:52 GMT
4) That's what I explained. 5) That is not what I said. There is your problem. However much trouble you're having with the math, you won't get over it till your English improves. What I did say is that the probability is 98/100 before they were opened. You're conflating the Monty Hall problem and the random "opening" or "elimination" of doors. Apparently Bayes' Theorem has not improved your ability to roll with the changes. 6) What I said. Sometimes when people get lost it helps to go to the top of the page and write everything out. Given: The probability the prize is behind exactly one of x (x = 3 e.g.) doors is a certainty or P=1. Given: With no further information the contestant selects one of the x doors. Given; The host knowing full well where the prize is opens or eliminates one of the two remaining doors giving the contestant new information. Solution: The probability the contestant chose the door with the prize is 1/x. (e.g. 1/3) The probability the prize is behind the remaining doors is 1 - 1/x. (e.g. 2/3) Notice that the sum of the probabilities is 1. That is important. See the first given. The contestant gets "new information" that the probability the prize is behind one of the remaining doors is zero, as it is opened or eliminated. However it is not new information to the person who selected it. The host already knew it was zero. This is the critical point to understand, and the reason some people have difficulty with the problem The sum of the probabilities must still add to 1. The probability the prize is behind the door the contestant selected is still 1/x. Since "we" (host, contestant and audience) have no new information. The probability it is behind the opened or eliminated remaining door is zero as we all now see. That leaves a probability of 1 - 1/x for the remaining closed door. For example Door 1 P = 1/x or 1/3, Door 2 P = 0, Door 3 P= 1 - 1/x or 2/3. For randomly selected "remaining" doors the probabilities must also add to 1.
The probability the host selects the right door is 1/x before any doors are opened. That leaves 1 - 1/x -1/x for the remaining door or if x = 3 then 1/3. A good question: if P = 1/3 for the contestant's door and P = 1/3 for the remaining door where is the other 1/3? That is in the times the host accidentally opens the door with the prize which is 1/3 of the time. That of course can only happen in this variation. I should also mention that if there are only two choices whose probability was determined to be the same are considered by themselves the probability becomes one half for each or "equal" probability. That change happens when you do not count the times the host accidentally opened the door with the prize. Another note: When I say the probability is 98/100 the prize is behind a door randomly opened that means that 98 percent of the time the host will accidentally open the door with the prize. Now you try it. Go to the top of the page and list line by line where you got your conditional and marginal probabilities and your 'A' and 'B' events. 4-6. You do realize your post is still there for everyone to read, yes? If so, I don't know why you just blatantly lied about what you said. What you actually said: "If 98 doors are randomly eliminated the chance the prize is behind the remaining door 1/100, the chance the prize is behind the first door picked is 1/100 and the chance the prize is not behind either remaining door is 98/100.." You most certainly DID NOT say that "the probability is 98/100 before they were opened." You also most certainly didn't say that "with randomness it's 50/50" because, again, I quote: "If we know the 98 eliminated doors do not have the prize then the chance the prize is behind the first door picked is still 1/100 and the chance the prize is behind the remaining doors is still 99/100 even if only one remains."
All I can surmise from this is that you realized from my post that you were wrong, and now you're gaslighting to try to convince people you didn't say exactly what I quoted you as having said. Everything else you said is just restating the same thing you did the first time around, that the "2/3 goes to the third door." Why does it do this? You've never explained why the 2/3 goes to the third door rather than to the first door, or just becomes 50/50. Saying that it is so is not an explanation. Bayes, as I used it, explained this using conditional reasoning. If you want it longhand (I've already done this before in the last thread, and you didn't get it then): P(A|B) = P(B|A)P(A) / (P(B|A)P(A)+P(B|~A)P(~A)) P(A|B) = Probability prize is behind D3 given Monty opened D2 P(B|A) = Probability Monty opened D2 given prize is behind D3 (1) P(A) = Probability prize is behind D3 (.33) P(B|~A) = Probability Monty opened D2 given prize isn't behind D3 (.5) P(~A) = Probability prize isn't behind D3 (.33) P(A|B) = 1*.33 / (1*.33 + .5*.33) P(A|B) = .33 / .5 P(A|B) = .67 I said doors were "eliminated" not "opened" and you should have gotten the meaning anyway from the context. Since you obviously forgot it, here's Bayes' Theorem. P(A|B) = ( P(B|A) x P(A) ) / ( P(B) )
|
|
|
Post by Eva Yojimbo on Feb 15, 2020 1:48:37 GMT
Depends on what you mean by "give it a different status." In what sense? If you just mean we consider it as such for its utility, then fine. I'm speaking more fundamentally, theoretically. I never denied the utility of using it. Ultimately, though, it relies on the assumption of truth of its propositions. I'm sure I don't have to tell you that in deductive logic propositions must be assumed true rather than proved themselves. If those assumptions rest on conclusions from other propositions, then that evidence must also rest on assumed propositions and so-on back to infinity. Any assumed truth could be wrong, even down to the most basic one that we can (generally) rely on our senses to tell us facts about reality. Plus, Hume knew long ago that you can't get from empirical evidence, which is inductive, to binary/universal truth values. To me, the easy way around this is to just say say that everything is really induction/probability, and deduction/Boolean truth values are useful fictions. I agree, of course, that the universe is disinterested in our estimations of how likely something is; that ignores the fact that we don't have direct access to how the universe is. Our only access is via our senses and reasoning, both of which can be fallible, both of which are limited. One reason science works is that it's able to update itself when new evidence is presented, but if we are absolutely, 100% certain that something's true then it would be impossible for new evidence to convince us otherwise. This should be an obvious indicator we never get to 100% certainty about anything. We just get awfully close sometimes. Both of your examples very much involve "probability assessment." In 1, there were hypotheses about Andromeda. Those hypotheses can be treated as our priors, which have some probability of being true. The invention of better telescopes provided new and better evidence as to which was true, and "galaxy" won out over "dust cloud" thanks to that evidence. In 2. you could say that "aether theory" was the prior hypothesis involved, that had some probability of being true, while the absence of evidence in things like the Michelson-Morley experiments was the evidence that aether wasn't true. This prompted Einstein to begin thinking about alternative explanations. This lead to the hypothesis of Relativity, a hypothesis that also had some probability of being true, which eventually lead to the various experiments which provided evidence that Relativity was very probably true. Again, if you really want to be convinced of this I'd recommend reading at least some of that Jaynes textbook. The biggest problem you're having is, again, not realizing that our hypotheses can be treated as priors, and experiments are the conditional probabilities that either provide evidence for them or not. What I mean by "give it a different status" is "give it a different status according to how fictional" is is. You described Boolean algebra as a "useful fiction". You could start talking about the useful fiction of integer math ("it's impossible to have exactly 3 bales of hay"). What really bugs me about Bayesian thinking is that it always goes on about priors. What difference does it make if ancient philosophers thought there was an 80 percent chance that the earth rode on the back of a giant tortoise, or some other number? When we gradually gained a more modern view of the nature of earth and space, we can just throw out the entirety of the old thinking (the baby with the bathwater) and any estimated probabilities (however formal, informal or implied). In my examples the point I'm making is that the crucial thinking process that led to the discovery, the breakthrough in insight wasn't an analysis of prior probabilities but of a measurement in one case and geometric insight in the other. It didn't matter if 60 percent or 90 percent of astronomers favored the dust cloud theory for Andromeda; once a scientific measurement was made it was clear who was right, and the prior probabilities became completely irrelevant. Einstein wasn't thinking at all of prior probabilities while doing his thought experiments. If you can give a satisfying answer to the last paragraph I may consider taking a look at Jaynes book, though the title of the book "Probability Theory" doesn't really suggest it would address these topics. Of course there are plenty of useful fictions out there, many of which are products of how we categorize reality mentality on higher levels of organization than what exists on some fundamental level. Temperature is another such useful fiction. So is (to quote Sean Carroll) baseball and free will. The importance of priors should be fairly obvious. Remember my coin-flip experiment thread? If you find a random coin and flip four heads in a row, the only reason you don't think it's more likely to be a trick coin immediately is your "prior" that fair coins are more common than trick coins. Otherwise, the "trick coin" hypothesis fits the data better than the "fair coin" hypothesis. The importance of priors is also good for explaining why design/intention isn't automatically the best explanation for any given phenomena. Essentially, how well a hypothesis "fits" the data is meaningless without knowing the likelihood of the hypothesis to begin with. I agree that as our evidence grows we're able to "throw out the entirety of the old thinking." What makes you think we can't/don't do that with priors? In science, the ideal we're striving for is to find an experiment that's able to elevate one hypothesis to ~100% and reduce all others to ~0%: "Given E(vidence), the P(robability) of H(ypothesis) is ~100%; Given E, the P of ~H is ~0%." Sometimes, as with Relativity, or Andromeda, we're able to find experiments that get awfully close to that ideal. Other times, as with evolution, say, it's more of a slow accumulation of evidence from multiple disciples over a long period of time. This is a way of saying that, yeah, those scientific experiments that can tell us which hypothesis is almost certain to be true are awesome; but in situations where we don't have that luxury, rationality is not powerless to help. A perfect example is with interpretations of quantum mechanics. All the experimental results can be explained equally well by all hypotheses/interpretations. The only difference is in how probable those hypotheses are to start with, and that's determined by how much they assume. The reason I've always argued that many-worlds is most likely is because every other interpretation is "many-worlds + other assumptions." According to the conjunction fallacy, that automatically makes every other interpretation less likely; and what is the impetus for adding non-mathematically justified, non-empirically justified, assumptions to ANY hypothesis/theory? Can you imagine a serious scientist saying "yeah, Maxwell's equations and Lorentz force work to explain electricity, but I also think there are hidden unicorns that are involved!" They'd be laughed out of science. Yet, for some bizarre reason, we can't apply that same reasoning to QM even though there's really no difference. The entire 20th century will be a history lesson for future generations as to what happens when scientists don't apply such basic rationality principles like Occam's Razor and prefer, instead, to find convoluted ways to justify their intuitions. Of course, it would be awesome if some scientist DID come along and find some experiment to PROVE many-worlds (or any other QM interpretation). They'd easily win the Nobel prize and it would likely be the biggest scientific breakthrough in a century. But it's rather stupid to think that, until we get that breakthrough, every interpretation/hypothesis is up for grabs, is equally likely. No, that's just stupid, and there's probably more situations in life where we simply DON'T have such definitive experiments that prove any given hypothesis.
|
|
|
Post by Eva Yojimbo on Feb 15, 2020 1:52:32 GMT
4-6. You do realize your post is still there for everyone to read, yes? If so, I don't know why you just blatantly lied about what you said. What you actually said: "If 98 doors are randomly eliminated the chance the prize is behind the remaining door 1/100, the chance the prize is behind the first door picked is 1/100 and the chance the prize is not behind either remaining door is 98/100.." You most certainly DID NOT say that "the probability is 98/100 before they were opened." You also most certainly didn't say that "with randomness it's 50/50" because, again, I quote: "If we know the 98 eliminated doors do not have the prize then the chance the prize is behind the first door picked is still 1/100 and the chance the prize is behind the remaining doors is still 99/100 even if only one remains."
All I can surmise from this is that you realized from my post that you were wrong, and now you're gaslighting to try to convince people you didn't say exactly what I quoted you as having said. Everything else you said is just restating the same thing you did the first time around, that the "2/3 goes to the third door." Why does it do this? You've never explained why the 2/3 goes to the third door rather than to the first door, or just becomes 50/50. Saying that it is so is not an explanation. Bayes, as I used it, explained this using conditional reasoning. If you want it longhand (I've already done this before in the last thread, and you didn't get it then): P(A|B) = P(B|A)P(A) / (P(B|A)P(A)+P(B|~A)P(~A)) P(A|B) = Probability prize is behind D3 given Monty opened D2 P(B|A) = Probability Monty opened D2 given prize is behind D3 (1) P(A) = Probability prize is behind D3 (.33) P(B|~A) = Probability Monty opened D2 given prize isn't behind D3 (.5) P(~A) = Probability prize isn't behind D3 (.33) P(A|B) = 1*.33 / (1*.33 + .5*.33) P(A|B) = .33 / .5 P(A|B) = .67 I said doors were "eliminated" not "opened" and you should have gotten the meaning anyway from the context. Since you obviously forgot it, here's Bayes' Theorem. P(A|B) = ( P(B|A) x P(A) ) / ( P(B) ) How in the world do you "eliminate" doors without opening them? You have to know what's behind them to eliminate them, and you have to open them to know what's behind them. I did not forget Bayes's Theorem. I gave the long version because last time you got confused over how I found P(B). The long version I gave above is identical to the version you gave, it just shows you how to derive P(B). See: en.wikipedia.org/wiki/Bayes%27_theorem#Alternative_form
|
|
|
Post by dividavi on Feb 15, 2020 2:05:01 GMT
Concerning the Monty Hall Problem, I think what Arlon10 is trying to establish is that there would be no apparent paradox if there were more than three doors to select. The show could have ten or a hundred doors with there being only one door hiding anything valuable. For a hundred door system the contestant would choose a door, say number 2, and Monty would eliminate 98 doors leaving only #2 and #71. Monty would ask if the contestant would prefer to change his guess and he would immediately do that. It's obvious that his initial guess had an overwhelming probablity (ninety nine in a hundred) of being wrong so he switches. Of course that would destroy the show but let's pretend that every contestant follows the procedure and changes the initial choice when called on to do so by Monty Hall. Every hundred shows, on average and assuming there's no cheating by the producers, somebody guesses correctly initially and changes his selection to something worthless. It'll happen, given time, and it'll happen more often with each reduction in the number of doors. For three doors, the contestant has probably made an initial incorrect choice with one-third probability of being right and two-thirds being wrong. It will quickly happen that a contestant who changes his selection will regret that change one out of three times. Those contestants will not wish to face the ridicule of their friends and family. "Why'd you change? You had the right choice to begin with." Better to lose "honorably", if that's the proper word.
|
|
|
Post by Eva Yojimbo on Feb 15, 2020 2:19:40 GMT
dividavi I absolutely agree that Arlon's 100-door example is a good way of getting people to intuitively understand the problem. My issue is that he still doesn't/can't explain WHY the remaining probability goes to the other door. You might say "but it's intuitively obvious that it does," which is fine, but isn't an actual explanation. To actually explain why, in a way that works for every variation (including Monty Fall and Monty Crawl) you need to understand conditional reasoning/probability, which isn't all that difficult to do. My problem with Arlon is that he's also incredulous Bayes can be used to solve it, even after I use Bayes to solve it (twice now; there was another thread on this), and even though it's often used as an example of how to apply Bayes in textbooks on probability.
|
|
|
Post by Arlon10 on Feb 15, 2020 2:40:28 GMT
I said doors were "eliminated" not "opened" and you should have gotten the meaning anyway from the context. Since you obviously forgot it, here's Bayes' Theorem. P(A|B) = ( P(B|A) x P(A) ) / ( P(B) ) How in the world do you "eliminate" doors without opening them? You have to know what's behind them to eliminate them, and you have to open them to know what's behind them. I did not forget Bayes's Theorem. I gave the long version because last time you got confused over how I found P(B). The long version I gave above is identical to the version you gave, it just shows you how to derive P(B). See: en.wikipedia.org/wiki/Bayes%27_theorem#Alternative_form P(A|B) = Probability prize is behind D3 given Monty opened D2 P(B|A) = Probability Monty opened D2 given prize is behind D3 (1) P(A) = Probability prize is behind D3 (.33) P(B|~A) = Probability Monty opened D2 given prize isn't behind D3 (.5) P(~A) = Probability prize isn't behind D3 (.33) < Oooops, in what scenario is that?
Now let's do 100 doors. P(A|B) = Probability prize is behind D100 given Monty opened D 2 through 99 P(B|A) = Probability Monty opened D 2 through 99 given prize is behind D100 (1) P(A) = Probability prize is behind D100 (1/100) < so far so good > P(B|~A) = Probability Monty opened D 2 through 99 given prize isn't behind D100 ( ??) Houston we have a problem P(~A) = Probability prize isn't behind D100 (99/100) Can you fix that? My method works plainly and simply for any number of doors. Yours appears it will take yet some more time. I'll use small words for your convenience Chance of picking 1 correct door out of 100 possible doors, very simply 1/100 Chance of correct door being in the other 99 doors, 1 - 1/100 = 99/100 Chance of one remaining door being correct after "opening" or "eliminating" the other 98 is still the same as when there were more doors because indeed the chance of those doors having the prize is and always was for the host zero. Totally Different
If the host randomly opens or eliminates doors it is obvious that there is a 98 percent chance he hits the door with the prize thus ruining the game 98 percent of the time (as I said and you failed to get) just as it would ruin the game 1/3 of the time with 3 doors. In such random scenarios the chance of the original and last doors having the prize is each 1/100 for 100 doors and each 1/3 for three doors, which is the even chance people often expect incorrectly in the Monty Hall problem. That the probabilities have to add to 1 is met when you add in the probability of accidentally opening the door with the prize and ruining the game. Edit > Obviously that could take a long time and he might need a programmable calculator. Meanwhile do you like cards? Lets use cards then. The host selects a card that represents the prize and makes a note somewhere hidden. The contestant then picks a card. The host then picks cards until only one is left being careful not to pick the card that is the prize. Please understand that the one card left by the host must be the prize in all circumstances except when the contestant chose the right card first. The math is very simple. The chance the last card left by the host is the prize is equal then to the chance the contestant chose the wrong card, which is 1 - 1/52 = 51/52. Obviously you can try this at home.
|
|
|
Post by Aj_June on Feb 15, 2020 18:01:23 GMT
Aj_June said: [ full text here] < clip >
Many of the biases that financial market participants display because of cognitive errors is linked to not updating views based on new information. Conservatism bias, in particular, is very strongly associated with a lack of rightful application of Bayes. Research has shown that quite a lot of investors suffer from biases such as conservatism and representativeness and that these biases often lead to overweighting prior beliefs while underweighting new information. I think it is important to note here that "probability" and "statistics" classes are not the same thing. Probability classes tend to deal with "known" probabilities and how to determine other probabilities from those, such as your taxicab problem does. Statistics classes tend to deal with the proper ways to gather data to arrive at any probability in the first place. The probabilities in the taxicab problem are simple to assess with considerable accuracy and there is little harm in granting them. (The color identification test is a little shaky and suspicious.) However most real life applications of probabilities are not so simple. It takes very careful data gathering techniques to avoid the multitude and magnitude of unknown and unmanageable variables as far as possible. Most often that is not very far. Of course and that was my point regarding how behavioural economics/ behavioural finance rebuts traditional economics and traditional finance. Most of the economic theories even now are based on the idea that human beings are totally rational and by extension the markets are efficient and rational at macro level. The concept of "rational economic man" is the bedrock of classical economics which says that: # Humans have perfect information about market prices # Humans make completely rational decisions to maximise their utility # Humans know what they want and efficiently make their choices. # Humans only act in self interest. Of course that is wrong because almost all humans suffer from cognitive biases to some degree, do not have perfect information and do make inefficient decisions. I personally do no not challenge the 4th point I listed and do believe classical economics is right about that. But behavioural economics/finance counters all those points. And one of the ways through which assumptions of classical economics is challenged is by demonstrating that humans suffer from biases which lead to them to making decisions which a perfectly rational person who acts on Bayes won't make.
|
|
|
Post by Arlon10 on Feb 15, 2020 18:58:32 GMT
I think it is important to note here that "probability" and "statistics" classes are not the same thing. Probability classes tend to deal with "known" probabilities and how to determine other probabilities from those, such as your taxicab problem does. Statistics classes tend to deal with the proper ways to gather data to arrive at any probability in the first place. The probabilities in the taxicab problem are simple to assess with considerable accuracy and there is little harm in granting them. (The color identification test is a little shaky and suspicious.) However most real life applications of probabilities are not so simple. It takes very careful data gathering techniques to avoid the multitude and magnitude of unknown and unmanageable variables as far as possible. Most often that is not very far. Of course and that was my point regarding how behavioural economics/ behavioural finance rebuts traditional economics and traditional finance. Most of the economic theories even now are based on the idea that human beings are totally rational and by extension the markets are efficient and rational at macro level. The concept of "rational economic man" is the bedrock of classical economics which says that: # Humans have perfect information about market prices # Humans make completely rational decisions to maximise their utility # Humans know what they want and efficiently make their choices. # Humans only act in self interest. Of course that is wrong because almost all humans suffer from cognitive biases to some degree, do not have perfect information and do make inefficient decisions. I personally do no not challenge the 4th point I listed and do believe classical economics is right about that. But behavioural economics/finance counters all those points. And one of the ways through which assumptions of classical economics is challenged is by demonstrating that humans suffer from biases which lead to them to making decisions which a perfectly rational person who acts on Bayes won't make. I do not remember there being any mention of "behavioral" economics in my economics classes. I do well remember though that we did study "perfect information." It is often assumed in simple modeling of markets and the assumption is sometimes far off. It was just one of quite many factors we studied that can throw the models off. We use models anyway as it can help understand the main factors. We did not assume that people maximize "utility." They probably do not. We did however assume they maximize what they believe they "want" or that they would make choices based on what they considered "best" for their personal tastes. Specifically they minimize "opportunity costs." Some people might wonder how they could possibly fail at minimizing opportunity costs, but there are those issues of perfect information and several other snags and obstacles. Another wonder is how people can possibly fail at acting in their own interests. If they "want" to help others, they have in fact made helping others, to whatever extent large or small, their own self interest. It's just playing with words to say they do not act in their own self interest. Of course government has the unique right to coerce and it can require things people would rather vote against. About the taxi problem now. I can understand how if ten people saw the same green taxi in poor light, two of them might say it's blue. That is because different people name their colors differently. I have difficulty accepting that one person saw the same green taxi in the same conditions and did not report the same color. Retail businesses often have electric signs that change colors and go off and on. That might explain how the same person could see the same green taxi ten times and report different colors. Otherwise it seems to me the witness either can or cannot distinguish the two colors. If he cannot then his guess should be wrong half the time. Am I correct that the taxi problem is just made up, not real? Students of statistics would red flag the claim of probability for the color identification test. They would require you catalog the retail business lights in the area and avoid errors based on those.
|
|
|
Post by Aj_June on Feb 15, 2020 19:57:10 GMT
Of course and that was my point regarding how behavioural economics/ behavioural finance rebuts traditional economics and traditional finance. Most of the economic theories even now are based on the idea that human beings are totally rational and by extension the markets are efficient and rational at macro level. The concept of "rational economic man" is the bedrock of classical economics which says that: # Humans have perfect information about market prices # Humans make completely rational decisions to maximise their utility # Humans know what they want and efficiently make their choices. # Humans only act in self interest. Of course that is wrong because almost all humans suffer from cognitive biases to some degree, do not have perfect information and do make inefficient decisions. I personally do no not challenge the 4th point I listed and do believe classical economics is right about that. But behavioural economics/finance counters all those points. And one of the ways through which assumptions of classical economics is challenged is by demonstrating that humans suffer from biases which lead to them to making decisions which a perfectly rational person who acts on Bayes won't make. I do not remember there being any mention of "behavioral" economics in my economics classes. I do well remember though that we did study "perfect information." It is often assumed in simple modeling of markets and the assumption is sometimes far off. It was just one of quite many factors we studied that can throw the models off. We use models anyway as it can help understand the main factors. We did not assume that people maximize "utility." They probably do not. We did however assume they maximize what they believe they "want" or that they would make choices based on what they considered "best" for their personal tastes. Specifically they minimize "opportunity costs." Some people might wonder how they could possibly fail at minimizing opportunity costs, but there are those issues of perfect information and several other snags and obstacles. Another wonder is how people can possibly fail at acting in their own interests. If they "want" to help others, they have in fact made helping others, to whatever extent large or small, their own self interest. It's just playing with words to say they do not act in their own self interest. Of course government has the unique right to coerce and it can require things people would rather vote against. About the taxi problem now. I can understand how if ten people saw the same green taxi in poor light, two of them might say it's blue. That is because different people name their colors differently. I have difficulty accepting that one person saw the same green taxi in the same conditions and did not report the same color. Retail businesses often have electric signs that change colors and go off and on. That might explain how the same person could see the same green taxi ten times and report different colors. Otherwise it seems to me the witness either can or cannot distinguish the two colors. If he cannot then his guess should be wrong half the time. Am I correct that the taxi problem is just made up, not real? Students of statistics would red flag the claim of probability for the color identification test. They would require you catalog the retail business lights in the area and avoid errors based on those. Behavioural finance/economics has emerged from behavioural psychology. You would not have studied that because it probably didn't exist in developed form when you studied economics, which might have been 40 years back.
|
|
|
Post by general313 on Feb 15, 2020 20:12:10 GMT
What I mean by "give it a different status" is "give it a different status according to how fictional" is is. You described Boolean algebra as a "useful fiction". You could start talking about the useful fiction of integer math ("it's impossible to have exactly 3 bales of hay"). What really bugs me about Bayesian thinking is that it always goes on about priors. What difference does it make if ancient philosophers thought there was an 80 percent chance that the earth rode on the back of a giant tortoise, or some other number? When we gradually gained a more modern view of the nature of earth and space, we can just throw out the entirety of the old thinking (the baby with the bathwater) and any estimated probabilities (however formal, informal or implied). In my examples the point I'm making is that the crucial thinking process that led to the discovery, the breakthrough in insight wasn't an analysis of prior probabilities but of a measurement in one case and geometric insight in the other. It didn't matter if 60 percent or 90 percent of astronomers favored the dust cloud theory for Andromeda; once a scientific measurement was made it was clear who was right, and the prior probabilities became completely irrelevant. Einstein wasn't thinking at all of prior probabilities while doing his thought experiments. If you can give a satisfying answer to the last paragraph I may consider taking a look at Jaynes book, though the title of the book "Probability Theory" doesn't really suggest it would address these topics. Of course there are plenty of useful fictions out there, many of which are products of how we categorize reality mentality on higher levels of organization than what exists on some fundamental level. Temperature is another such useful fiction. So is (to quote Sean Carroll) baseball and free will. I'll try to keep that in mind next time I forget to put in winter window-washer fluid in my car and I lose the ability to clean my windshield. I haven't said that priors aren't important in some cases, and your coin-flip experiment is a good example. Cladistics and other sciences involving DNA matching would fall in this category. But in those others where we throw out the entirety of the old thinking, including the priors, then we are no longer using anything like Bayes's theorem, except perhaps a trivial reduction of it. To capture Einstein's imagination in his relativity thought experiments, you have to look elsewhere than Bayes. QM is different than Maxwell's equations (even when viewing the latter from the perspective of the 19th century) because it's so bizarre and counter-intuitive. It may be urban legend that Feynman said "Anyone who claims to understand quantum theory is either lying or crazy" but I think there's a lot of truth in it regardless of whether it's an accurate quote. Until scientists develop some kind of experiment that can shed light on some of these interpretations it remains beyond the realm of science. I'm a great fan of Occam's Razor but it shouldn't be confused as evidence, rather it's a good guide when we lack actual evidence. The atom has turned out to be much more complicated than Rutherford anticipated, so nature isn't always as simple as we would like it to be (at some particular moment). Meanwhile, that these interpretations remain unresolved isn't stopping semiconductor device engineers from building faster and better chips.
|
|
|
Post by Eva Yojimbo on Feb 16, 2020 2:20:49 GMT
Of course there are plenty of useful fictions out there, many of which are products of how we categorize reality mentality on higher levels of organization than what exists on some fundamental level. Temperature is another such useful fiction. So is (to quote Sean Carroll) baseball and free will. I'll try to keep that in mind next time I forget to put in winter window-washer fluid in my car and I lose the ability to clean my windshield. I haven't said that priors aren't important in some cases, and your coin-flip experiment is a good example. Cladistics and other sciences involving DNA matching would fall in this category. But in those others where we throw out the entirety of the old thinking, including the priors, then we are no longer using anything like Bayes's theorem, except perhaps a trivial reduction of it. To capture Einstein's imagination in his relativity thought experiments, you have to look elsewhere than Bayes. QM is different than Maxwell's equations (even when viewing the latter from the perspective of the 19th century) because it's so bizarre and counter-intuitive. It may be urban legend that Feynman said "Anyone who claims to understand quantum theory is either lying or crazy" but I think there's a lot of truth in it regardless of whether it's an accurate quote. Until scientists develop some kind of experiment that can shed light on some of these interpretations it remains beyond the realm of science. I'm a great fan of Occam's Razor but it shouldn't be confused as evidence, rather it's a good guide when we lack actual evidence. The atom has turned out to be much more complicated than Rutherford anticipated, so nature isn't always as simple as we would like it to be (at some particular moment). Meanwhile, that these interpretations remain unresolved isn't stopping semiconductor device engineers from building faster and better chips. The point with temperature is that it doesn't exist on any fundamental level of physics. Rather, it's an estimation of the average kinetic energy within a system. Break that down and all that actually exists is lots of particles moving at certain speeds. The notion of "average" and what's considered a "system" are ways in which our brain categorizes things on larger levels than the fundamental one. They're mental concepts about reality not found in fundamental descriptions of reality: "useful fictions." You do realize that once evidence modifies a prior the old prior essentially "doesn't exist" anymore, yes? All you're doing is using a kind of hindsight bias to say the prior never mattered, and that's just silly. Yeah, once we find an experiment that provides us the "Given E(vidence), the P(robability) of H(ypothesis) is ~100%; Given E, the P of ~H is ~0%." evidence then the old prior doesn't matter because the new evidence overwhelms whatever it is. I'd argue that's neither the situation in the vast majority of science, nor the vast situations in everyday life. Nearly everything you do in your everyday life is not a product of new evidence provided by some definitive experiment, but is a product of priors built from past evidence. If you walk out your door without fearing an airplane will fall on your head, that's because your "prior" experience of planes not falling on your head, not because of some rigorous new experiment you did before you walked out your door to prove a plane won't fall on your head. If you were to come home and find your home ransacked and things gone, your first instinct is "human burglars" not "alien burglars," and that's a product of priors, not some rigorous investigation to rule out aliens. I could come up with a near infinite examples of why our priors are crucially important in everyday life. Besides, even experiments of the kind that proved Relativity are STILL operating on Bayes. That "Given E(vidence), the P(robability) of H(ypothesis) is ~100%; Given E, the P of ~H is ~0%" is still Bayesian in nature. QM is "bizarre and counter-intuitive" when you try to force our intuitions about how the world is onto QM rather than just taking what QM say is happening seriously. It's amazing how science has such a history of shattering human intuitions, yet here are in the 21st century and scientists, the very people using the discipline that's supposed to minimize human biases, are still clinging to those biases and intuitions for no good reason when the math, and what it says about reality, are staring us in the face. Occam's razor is more than just "a guide," it's a mathematically provable notion that simpler hypotheses are more probable than others (look up Solomonoff Induction). The point is, in QM we have a model that perfectly describes how particles behave. We call it the "wavefunction" (or Shrodinger's Wave Equation). If we take that as it is, there are many-worlds. Simple as that. What science has done in the 20th century have added things to that to make the "many-worlds" go away. Not because an experiment says they don't exist (experiments say they do), not because they math says they don't exist (the math says they do), but because they don't like them intuitively. Sorry, but that's stupid, and it's not less stupid because it's fueled by bias and intuition, and it's not less stupid than adding unicorns to electricity.
|
|
|
Post by Eva Yojimbo on Feb 16, 2020 3:02:21 GMT
How in the world do you "eliminate" doors without opening them? You have to know what's behind them to eliminate them, and you have to open them to know what's behind them. I did not forget Bayes's Theorem. I gave the long version because last time you got confused over how I found P(B). The long version I gave above is identical to the version you gave, it just shows you how to derive P(B). See: en.wikipedia.org/wiki/Bayes%27_theorem#Alternative_form P(A|B) = Probability prize is behind D3 given Monty opened D2 P(B|A) = Probability Monty opened D2 given prize is behind D3 (1) P(A) = Probability prize is behind D3 (.33) P(B|~A) = Probability Monty opened D2 given prize isn't behind D3 (.5) P(~A) = Probability prize isn't behind D3 (.33) < Oooops, in what scenario is that? P(~A) is the scenario in which the prize is behind D1, the only other remaining possibility. Should be fairly obvious. Now let's do 100 doors. P(A|B) = Probability prize is behind D100 given Monty opened D 2 through 99 P(B|A) = Probability Monty opened D 2 through 99 given prize is behind D100 (1) P(A) = Probability prize is behind D100 (1/100) < so far so good > P(B|~A) = Probability Monty opened D 2 through 99 given prize isn't behind D100 ( ??) Houston we have a problem P(~A) = Probability prize isn't behind D100 (99/100) Can you fix that? Yes, I can fix that. P(B|~A) is .01. If the prize is behind D1 (which is another way of saying "not behind D100" as there are only two possibilities), Monty had a 1/99 chance of opening all doors besides D100. P(~A) is also .01 if we're going with the original probability, not .99 as you suggested here. Here's full Bayes for your 100-door variation: P(A|B) = Probability prize is behind D100 given Monty opened D2-99 P(B|A) = Probability Monty opened D2-99 given prize is behind D100 (1) P(A) = Probability prize is behind D100 (.01) P(B|~A) = Probability Monty opened D2-99 given prize isn't behind D100 (.01) P(~A) = Probability prize isn't behind D100 (.01) P(A|B) = 1*.01 / (1*.01 + .01*.01) P(A|B) = .01 / .0101 P(A|B) = .99 One thing I think you're getting confused about is thinking P(~A) must be 1-A and that's not necessary for Bayes to work. As long as A and ~A have the same correct ratio as "A" and "1-A," Bayes still works. So in the Monty Hall case you can use .5/.5, or you can use .33/.33, or 1/1, or .01/.01 and still get the same (correct) answer. What matters is getting the correct ratio. In the three door version I like to use the original .33/.33 as I just find it more intuitive to stick with the original probabilities, but you could also use .5/.5. Same in the 100 door version, you can use .5/.5 for the remaining two doors, or .01/.01. My method works plainly and simply for any number of doors. Yours appears it will take yet some more time. Your method can't account for variations. When I ask "why does the remaining probability go to the remaining door, rather than the chose door, or split evenly between them?" Your response that "it just does" is not an answer. Conditional probabilities/reasoning is the "why." If the host randomly opens or eliminates doors it is obvious that there is a 98 percent chance he hits the door with the prize thus ruining the game 98 percent of the time (as I said and you failed to get) just as it would ruin the game 1/3 of the time with 3 doors. In such random scenarios the chance of the original and last doors having the prize is each 1/100 for 100 doors and each 1/3 for three doors, which is the even chance people often expect incorrectly in the Monty Hall problem. That the probabilities have to add to 1 is met when you add in the probability of accidentally opening the door with the prize and ruining the game. Edit > Obviously that could take a long time and he might need a programmable calculator. Meanwhile do you like cards? Lets use cards then. The host selects a card that represents the prize and makes a note somewhere hidden. The contestant then picks a card. The host then picks cards until only one is left being careful not to pick the card that is the prize. Please understand that the one card left by the host must be the prize in all circumstances except when the contestant chose the right card first. The math is very simple. The chance the last card left by the host is the prize is equal then to the chance the contestant chose the wrong card, which is 1 - 1/52 = 51/52. Obviously you can try this at home. I obviously agree that in the random scenario the 100-door game is ruined 98% of the time and the 3-door game 33% of the time, but I was going off what YOU said. YOU said the host randomly opened 98 doors. I'm sorry if you messed up your own word problem. I also agree the probability is 50/50 in any random scenario. But, again, you just saying "it is" is not an answer as to why it is. Again, conditional reasoning/probability explains why it is. Same thing with the card scenario, you saying "The chance the last card left by the host is the prize is equal then to the chance the contestant chose the wrong card" is not an explanation as to why that is so. Conditional reasoning/probabilities explain why it is so. Do I need to do Bayes again? Or have you gotten it by now?
|
|
|
Post by Arlon10 on Feb 16, 2020 4:33:48 GMT
Eva Yojimbo said: [ full text here] < clips >
1) P(B|~A) = Probability Monty opened D2-99 given prize isn't behind D100 (.01) 2) P(~A) = Probability prize isn't behind D100 (.01) 3) Your method can't account for variations. 4) you just saying "it is" is not an answer as to why it is I'm sorry, this is taking more time than I have the interest. On a cursory inspection it appears you're getting the right answer by using my methods then making up values to plug into Bayes' Theorem to get that answer with little justification in the theorem. Your choice of .01 in "1)" is you "just saying it is" as you accuse me in "4)." I believe the story is much longer. I still think your problem is English, not math, thus I can give you some benefit of the doubt. Here is what I consider an English sentence that leads to a formula. "Understand that the one card left by the host must be the prize in all circumstances except when the contestant chose the right card first" is plain English for 'A' and '~A.' Since the chance of the contestant getting the right card/door on first choice is 1/n where n = number of doors/cards and "all circumstances" here means a probability of one, the formula for the probability of the last card/door being the prize is very simply 1 - 1/n. An English approach if you did not get that would be to say this. When the contestant chooses a card it is like they are face down whether they are or not because he has no idea which one has been designated as the prize. When the host chooses cards they are face up. He can see if the prize card is still in the deck and not choose it till it is the only one left. Therefore it has to be the only one left unless the contestant chose it in the first place.
|
|
|
Post by goz on Feb 16, 2020 5:28:22 GMT
Eva Yojimbo said: [ full text here] < clips >
1) P(B|~A) = Probability Monty opened D2-99 given prize isn't behind D100 (.01) 2) P(~A) = Probability prize isn't behind D100 (.01) 3) Your method can't account for variations. 4) you just saying "it is" is not an answer as to why it is I'm sorry, this is taking more time than I have the interest. On a cursory inspection it appears you're getting the right answer by using my methods then making up values to plug into Bayes' Theorem to get that answer with little justification in the theorem. Your choice of .01 in "1)" is you "just saying it is" as you accuse me in "4)." I believe the story is much longer. I still think your problem is English, not math, thus I can give you some benefit of the doubt. Here is what I consider an English sentence that leads to a formula. "Understand that the one card left by the host must be the prize in all circumstances except when the contestant chose the right card first" is plain English for 'A' and '~A.' Since the chance of the contestant getting the right card/door on first choice is 1/n where n = number of doors/cards and "all circumstances" here means a probability of one, the formula for the probability of the last card/door being the prize is very simply 1 - 1/n. An English approach if you did not get that would be to say this. When the contestant chooses a card it is like they are face down whether they are or not because he has no idea which one has been designated as the prize. When the host chooses cards they are face up. He can see if the prize card is still in the deck and not choose it till it is the only one left. Therefore it has to be the only one left unless the contestant chose it in the first place. Yes, the English is often tricky to understand. Give the contestant some slack... It is really hard to pick the right card when you are face down!  especially when the host chooses the cards...
|
|
|
Post by Eva Yojimbo on Feb 16, 2020 6:12:18 GMT
Eva Yojimbo said: [ full text here] < clips >
1) P(B|~A) = Probability Monty opened D2-99 given prize isn't behind D100 (.01) 2) P(~A) = Probability prize isn't behind D100 (.01) 3) Your method can't account for variations. 4) you just saying "it is" is not an answer as to why it is I'm sorry, this is taking more time than I have the interest. On a cursory inspection it appears you're getting the right answer by using my methods then making up values to plug into Bayes' Theorem to get that answer with little justification in the theorem. Your choice of .01 in "1)" is you "just saying it is" as you accuse me in "4)." I believe the story is much longer. I still think your problem is English, not math, thus I can give you some benefit of the doubt. Here is what I consider an English sentence that leads to a formula. "Understand that the one card left by the host must be the prize in all circumstances except when the contestant chose the right card first" is plain English for 'A' and '~A.' Since the chance of the contestant getting the right card/door on first choice is 1/n where n = number of doors/cards and "all circumstances" here means a probability of one, the formula for the probability of the last card/door being the prize is very simply 1 - 1/n. An English approach if you did not get that would be to say this. When the contestant chooses a card it is like they are face down whether they are or not because he has no idea which one has been designated as the prize. When the host chooses cards they are face up. He can see if the prize card is still in the deck and not choose it till it is the only one left. Therefore it has to be the only one left unless the contestant chose it in the first place. It's amazing you always start running out of "interest" when someone is proving you wrong. You suffered the same sudden "lack of interest" the last time we discussed this at precisely this same point. Your "cursory inspection" is wrong. P(B|~A) is the probability Monty randomly opened every door except D100. Another way to ask that question is "what's the probability Monty randomly leaves ANY door unopened?" The answer is 1/99, or .01. Again, my issue with your 100-door variation, or 52-card variation, isn't that you can't get the right answer and intuitively understand why that is; my problem is that you can't explain why that is. You just keep repeating "it is so" without explaining why. What's so hard/complicated about saying: "Given the right card/door is my original choice (.02/.01, respectively), the probability host eliminated 50 cards/98 doors leaving that card/door is .02/.01, respectively; given the right card/door is the the last choice (.02/.01, respectively), the probability the host eliminated 98 doors/50 cards leaving that door/card is 1." Here's the difference in our views, as I see it. You're wanting to start from a position of saying the probability the prize is behind D1 is 1%, the probability it's in D2-100 is 99%, and when we eliminate D2-99 the 99% just "transfers" to D100. This isn't what happens. What happens is that after D2-99 are eliminated you have a 50/50 shot given no additional information; but the intention (or lack of intention) behind the host's choice IS additional information. That additional information is modeled when we think conditionally: If I chose right, the host randomly opened doors, and the probability of him randomly leaving only D100 is 1/99. If I chose wrong, the host DIDN'T randomly open doors, and the probability of him only leaving D100 closed is 1. You just gloss over this reasoning in your version. I don't know why you're doing this other than that you don't understand it.
|
|
|
Post by Arlon10 on Feb 16, 2020 13:20:53 GMT
I'm sorry, this is taking more time than I have the interest. On a cursory inspection it appears you're getting the right answer by using my methods then making up values to plug into Bayes' Theorem to get that answer with little justification in the theorem. Your choice of .01 in "1)" is you "just saying it is" as you accuse me in "4)." I believe the story is much longer. I still think your problem is English, not math, thus I can give you some benefit of the doubt. Here is what I consider an English sentence that leads to a formula. "Understand that the one card left by the host must be the prize in all circumstances except when the contestant chose the right card first" is plain English for 'A' and '~A.' Since the chance of the contestant getting the right card/door on first choice is 1/n where n = number of doors/cards and "all circumstances" here means a probability of one, the formula for the probability of the last card/door being the prize is very simply 1 - 1/n. An English approach if you did not get that would be to say this. When the contestant chooses a card it is like they are face down whether they are or not because he has no idea which one has been designated as the prize. When the host chooses cards they are face up. He can see if the prize card is still in the deck and not choose it till it is the only one left. Therefore it has to be the only one left unless the contestant chose it in the first place. It's amazing you always start running out of "interest" when someone is proving you wrong. You suffered the same sudden "lack of interest" the last time we discussed this at precisely this same point. Your "cursory inspection" is wrong. P(B|~A) is the probability Monty randomly opened every door except D100. Another way to ask that question is "what's the probability Monty randomly leaves ANY door unopened?" The answer is 1/99, or .01. Again, my issue with your 100-door variation, or 52-card variation, isn't that you can't get the right answer and intuitively understand why that is; my problem is that you can't explain why that is. You just keep repeating "it is so" without explaining why. What's so hard/complicated about saying: "Given the right card/door is my original choice (.02/.01, respectively), the probability host eliminated 50 cards/98 doors leaving that card/door is .02/.01, respectively; given the right card/door is the the last choice (.02/.01, respectively), the probability the host eliminated 98 doors/50 cards leaving that door/card is 1." Here's the difference in our views, as I see it. You're wanting to start from a position of saying the probability the prize is behind D1 is 1%, the probability it's in D2-100 is 99%, and when we eliminate D2-99 the 99% just "transfers" to D100. This isn't what happens. What happens is that after D2-99 are eliminated you have a 50/50 shot given no additional information; but the intention (or lack of intention) behind the host's choice IS additional information. That additional information is modeled when we think conditionally: If I chose right, the host randomly opened doors, and the probability of him randomly leaving only D100 is 1/99. If I chose wrong, the host DIDN'T randomly open doors, and the probability of him only leaving D100 closed is 1. You just gloss over this reasoning in your version. I don't know why you're doing this other than that you don't understand it. Have you tried pressing CTRL+ALT+DEL ? I know I have, and still no explanations from you. Be forewarned, improving your English won't help in cases where people do not want to understand what you say. I have a much lower tolerance for needless repetition than most people here. That's all. Try a key or rhythm shift to keep it interesting.
|
|
|
Post by Arlon10 on Feb 16, 2020 14:03:21 GMT
I'm sorry, this is taking more time than I have the interest. On a cursory inspection it appears you're getting the right answer by using my methods then making up values to plug into Bayes' Theorem to get that answer with little justification in the theorem. Your choice of .01 in "1)" is you "just saying it is" as you accuse me in "4)." I believe the story is much longer. I still think your problem is English, not math, thus I can give you some benefit of the doubt. Here is what I consider an English sentence that leads to a formula. "Understand that the one card left by the host must be the prize in all circumstances except when the contestant chose the right card first" is plain English for 'A' and '~A.' Since the chance of the contestant getting the right card/door on first choice is 1/n where n = number of doors/cards and "all circumstances" here means a probability of one, the formula for the probability of the last card/door being the prize is very simply 1 - 1/n. An English approach if you did not get that would be to say this. When the contestant chooses a card it is like they are face down whether they are or not because he has no idea which one has been designated as the prize. When the host chooses cards they are face up. He can see if the prize card is still in the deck and not choose it till it is the only one left. Therefore it has to be the only one left unless the contestant chose it in the first place. Yes, the English is often tricky to understand. Give the contestant some slack... It is really hard to pick the right card when you are face down!  especially when the host chooses the cards... The neighboring antecedent of "they" is "card" and the logical antecedent of "they" is "card." I consider this board a "casual" setting. For more formality, "When the contestant chooses a card it is like they the cards are face down. Happy now? I know "they" is used with a singular antecedent often these days and it annoys me too. People use "they" for a singular antecedent that cannot be identified as male or female, as in, "When a person (singular) registers to vote they (plural) must bring two forms of identification." That is done because "he" or "she" is wrong and "he or she" is long and awkward, or at least considered awkward. Another solution is, "When people (plural) register to vote they (plural) must bring two forms of identification." A tiny problem with that is people don't register to vote as a crowd, the register to vote as individuals. In my further defense, it is a "card" when picked but from the deck of "cards" that is face down.
|
|
|
Post by Eva Yojimbo on Feb 16, 2020 14:19:47 GMT
It's amazing you always start running out of "interest" when someone is proving you wrong. You suffered the same sudden "lack of interest" the last time we discussed this at precisely this same point. Your "cursory inspection" is wrong. P(B|~A) is the probability Monty randomly opened every door except D100. Another way to ask that question is "what's the probability Monty randomly leaves ANY door unopened?" The answer is 1/99, or .01. Again, my issue with your 100-door variation, or 52-card variation, isn't that you can't get the right answer and intuitively understand why that is; my problem is that you can't explain why that is. You just keep repeating "it is so" without explaining why. What's so hard/complicated about saying: "Given the right card/door is my original choice (.02/.01, respectively), the probability host eliminated 50 cards/98 doors leaving that card/door is .02/.01, respectively; given the right card/door is the the last choice (.02/.01, respectively), the probability the host eliminated 98 doors/50 cards leaving that door/card is 1." Here's the difference in our views, as I see it. You're wanting to start from a position of saying the probability the prize is behind D1 is 1%, the probability it's in D2-100 is 99%, and when we eliminate D2-99 the 99% just "transfers" to D100. This isn't what happens. What happens is that after D2-99 are eliminated you have a 50/50 shot given no additional information; but the intention (or lack of intention) behind the host's choice IS additional information. That additional information is modeled when we think conditionally: If I chose right, the host randomly opened doors, and the probability of him randomly leaving only D100 is 1/99. If I chose wrong, the host DIDN'T randomly open doors, and the probability of him only leaving D100 closed is 1. You just gloss over this reasoning in your version. I don't know why you're doing this other than that you don't understand it. Be forewarned, improving your English won't help in cases where people do not want to understand what you say. No kidding. I know you've perfected the art of not wanting to understand what others say when it would require admitting you were wrong.
|
|
|
Post by Arlon10 on Feb 16, 2020 14:41:14 GMT
Be forewarned, improving your English won't help in cases where people do not want to understand what you say. No kidding. I know you've perfected the art of not wanting to understand what others say when it would require admitting you were wrong. Memories.
|
|